Microelectronics Institute and others have made progress in energy-efficient floating-point in-memory computing
In recent years, in-memory computing architecture has become an important research direction for artificial intelligence acceleration chips. The in-memory computing architecture can reduce data access overhead and significantly improve the energy efficiency of intelligent algorithms such as neural networks. At present, research on in-memory computing chips is concentrated in the field of fixed-point in-memory computing. Existing implementations of floating-point in-memory computing use near-memory circuits, exponent-base separation, floating-point-fixed-point conversion, etc. , facing low parallelism Or the challenge of more floating-point operation cycles, it is difficult to achieve high energy efficiency and high performance. Considering the needs of larger-scale network models and complex tasks, as well as the necessity of floating-point operations in the neural network training process, the floating-point operation function is very important for future in-memory computing chips.
Aiming at the challenge of energy-efficient floating-point in-memory computing, the team of Liu Ming, academician of the Chinese Academy of Sciences and researcher at the Institute of Microelectronics of the Chinese Academy of Sciences, and the team of Professor Liu Yongpan of Tsinghua University have proposed a new hybrid in-memory computing architecture of " dense memory + sparse numbers " . The study found that the data distribution of each layer of the neural network has a long-tail characteristic, and the exponential bits of most of the data are densely distributed in a small interval. Based on this, the research proposes to use the high-efficiency in-memory computing core to perform data operations with dense exponent bits, and use the highly flexible sparse digital core to perform data operations with sparse exponent bits with long-tail characteristics. High-efficiency floating -point - to-fixed-point conversion circuits, a flexible-coded sparse digital core, and an adder tree circuit for digital in-memory computations that save high-bit computations further enhance the energy efficiency of the design. The SRAM in-memory computing chip is taped out under the 28nm process. In the case of 4- bit specific point and 16- bit floating point, the peak energy efficiency of the dense network of the in-memory computing core reaches 275TOPS/W and 17.2TOPS/W , respectively, and the peak energy efficiency of the sparse network reaches 1600TOPS/W and 90TOPS/W . This achievement will help promote the application of SRAM in-memory computing chips in high-precision floating-point neural networks and neural network training.
The relevant research results are titled
A 28nm 16.9-300TOPS/W Computing-in-Memory Processor Supporting Floating-Point NN Inference/Training with Intensive-CIM Sparse-Digital Architecture , which was selected into the top conference ISSCC 2023 in the field of solid-state circuits .
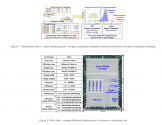