Deepseek r1 weights released...
This is like dropping an atomic warhead on OpenAI
Mother of God this one is stronk... beats o1 medium....
I have read the paper
.
It is incredibly interesting, especially the part about RL (reinforcement learning) that is what enables the "reasoning". RL is a vast and active research field and there are many RL recipes that researchers are continuously inventing/testing.
Deepseek guys are the first ones with a SOTA reasoning model to openly show their recipe.
Their recipe is called
Group Relative Policy Optimization (GRPO). Is their independent invention, an improvement above
invented 1.5 years ago that is itself an improvement over
(2017).
RL improves a model by forcing it to maximize an objective. It is not a "next token prediction", but quite a more complex one. In case of this new model is:
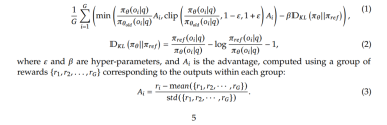
This seems a bit intimidating, but actually is not.
I will try to explain in simple terms because what we are witnessing here is IMHO the beginning of something big.
To teach their model to reasoning they do the following:
1.
They sample N answers for each question: They ask the same question to the model N times and get N answers, every time a different one.
2. They compute the
probability of each answer as the product of the probability of each token (word) given the previous ones. For instance, remembering that LLMs generate one token at a time, if the question + current partial generated answer is "What is the capital of US? The capital of " They read and store the probability of generating "US" token (probability for each emitted token is always computed by the LLM as part of its generation process). They continue until the end of "question + answer" sentence.
3. They
reward the answers, i.e. they verify if answer is correct or not. Assume the reward is 1 for correct answer and 0 for incorrect answer and LLM generates 10 responses: 3 correct and 7 wrong: the mean reward will be 0.3 (i.e. 3/10)
4. They
compute the Advantage (eq 3) that is simply subtracting the mean for each answer reward (ignore the std deviation at denominator). So correct answers will have advantage of 1 - 0.3 = 0.7, while wrong answers will have advantage 0 -0.3 = -0.3 (here negative is crucial!)
5. They
compute the objective to maximize as sums of this term (ignore clipping and the last Dkl term are needed only to give stability to training):
Here the pi terms at numerator and denominator are the new optimized LLM and the old LLM from which training epoch start. Let's assume they are the same. So that term means the probability that the LLM, given the question q ("What is the capital of US?") answers with answer Oi (our i-th answer out of 10 we got before). Crucially that term is multiplied by the advantage Ai (i.e. 0.7 if answer ok or -0.3 if answer wrong). The first 1/G followed by big E it just means the sum of each term, one for each answer, so 10 in total. Divided by 10 (i.e. the average).
So the quantity that LLM training has to maximizes is like: 0.7*probability(answer 1) -0.3*prob(a2) +.....+ 0.7*prob(a10)
We can see immediately that any change in LLM weights that improves probability of a correct answer increases the objective, while those that improve probability of a wrong answer decrease it (due to negative advantage coefficient).
The RL training just makes thousands of questions and sample many more answers, compute the above term and updates the LLM weights accordingly and slowly but surely it seems that...it works!
One critical observation is that
only the full answer is rewarded, not every token of it. IOW the
LLM is free to think whatever it wants as long as the result is ok. There is no external constrain on the thinking process.
And with great surprise of researchers, during the middle of training the LLM started to "evolve" in a totally independent and autonomous way:
